Hi
I have treatment versus control collected in eight batches (NanoString transcriptome)
I have tried NanoStringNCTools and RUVseq to remove unwanted variations
Here I suppose my eight batches can introduce unwanted technical variations
I have twenty housekeeping genes in my panel using them I want to combat with unwanted variations
So,
## ----ruv_spikes, fig.cap="RUVg normalization based on spike-in controls.", fig.subcap=c("RLE plot","PCA plot")----
set1 <- RUVg(set, spikes, k=1)
pData(set1)
> set1$x
[1] 1 1 1 1 1 1 1 1 1 2 2 2 2 2 2 2 2 2 2 2 2 3 3 3 3 3 3 4
[29] 4 4 4 4 4 4 4 4 4 4 4 5 5 5 5 5 5 5 5 5 5 5 5 6 6 6 7 7
[57] 7 7 7 7 7 7 8 8 8 8 8 8 8 8 8 8 8
Levels: 1 2 3 4 5 6 7 8
Please ,if you just tell me what W_1
is embedded in set1?
I know x is the main design ; But I am sure W_1 comes from where?
Is that correcting factor based on spikes in gene?
For instance as a part of tutorial you mention
This is done by considering a design matrix that includes both the covariates of interest (here, the treatment status) and the factors of unwanted variation.
design <- model.matrix(~x + W_1, data=pData(set1))
I have both covariate of interest and some some batches, so shall I still include the batch in the formula?
What would be a formula comparing treatment/control, removing unwanted variation and deducing batches?
I am desperately confused
Thanks for any clarification
Thanks a million
I have done so
I have plotted PCA for different things in my colData
The number of batches we collected the data before using housekeeping genes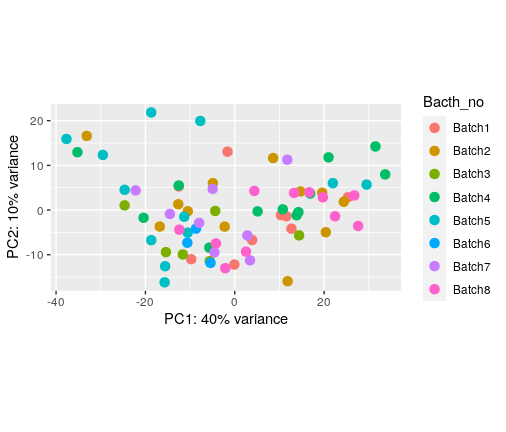
The number of batches we collected the data after using housekeeping genes (control genes)
And the other features (my main interest in Status_2 thought)